Enhancing Conveyor System Reliability with Machine Learning at Thiele.
Enhancing Conveyor System Reliability with Machine Learning at Thiele.
Estimated Gains
Projected Revenue Increase: €14.3M by 2027 (Estimate)
Cost Savings
Anticipated Operational Cost Savings: €4M (2024-2027, Estimate)
Revenue Increase
Forecasted 8% Annual Revenue Growth (Estimate)
IRR
Internal Rate of Return: Projected: 103.07%
Introduction
Thiele GmbH & Co. KG, a leading manufacturer specializing in chains and conveyor technology, faced significant challenges due to breakdowns in their conveyor systems, which are critical in industries such as mining and chemicals. In response, I led a strategic initiative that involved an in-depth analysis and the development of a machine learning (ML) solution to predict and prevent equipment failures, enhancing Thiele’s product reliability and operational efficiency. Drawing upon insights from Boston Consulting Group, which notes that ’successful digital transformations in the mining industry can lead to improvements in mining throughput by 10% to 20%, procurement productivity by up to 50%, and reduced emissions by 15% to 30%‘ (Ganeriwalla et al., 2021), our approach aimed to strategically align Thiele with these potential gains.
Strategic Overview
Incorporating Advanced ML for Predictive Maintenance
The project was initiated following consecutive equipment failures at a key customer’s plant, which highlighted the urgent need for a predictive maintenance strategy. My role involved analyzing the existing data, understanding the operational challenges, and spearheading the development of an ML model capable of anticipating equipment malfunctions before they occur.
Technical Implementation
Deploying Machine Learning to Drive Operational Excellence
I collaborated with Thiele’s engineering team and external partners, including IconPro and Talpa Solutions, to integrate unsupervised and supervised learning algorithms. This initiative aimed to leverage vast amounts of operational data from IoT sensors to forecast potential failures, thereby reducing downtime and maintenance costs.
Outcomes and Business Impact
Realizing the Value of Predictive Analytics in Heavy Industry
The implementation of the ML model marked a significant advancement in Thiele’s maintenance strategy. It allowed for real-time monitoring and prediction of system health, significantly reducing unplanned downtimes and associated costs. This shift not only safeguarded critical mining operations but also solidified Thiele’s reputation as a forward-thinking leader in conveyor technology.
Future Directions
Sustaining Innovation and Continuous Improvement
Building on the success of the initial ML deployment, Thiele is now exploring additional enhancements, including the integration of more complex sensors and expanding ML capabilities across other product lines. This ongoing innovation is aligned with Thiele’s strategic objective to provide cutting-edge, reliable solutions to the bulk goods transportation industry.
References
Ganeriwalla, A., Harnathka, S., Costa, A., Volkov, M., & Voigt, N. (2021). Racing toward a digital future in metals and mining. Boston Consulting Group. Available at: https://web-assets.bcg.com/65/43/b63e686f453d82fb46f6a0702e2d/bcg-racing-toward-a-digital-future-in-metals-and-mining-feb-2021.pdf [Accessed 16 April 2023].
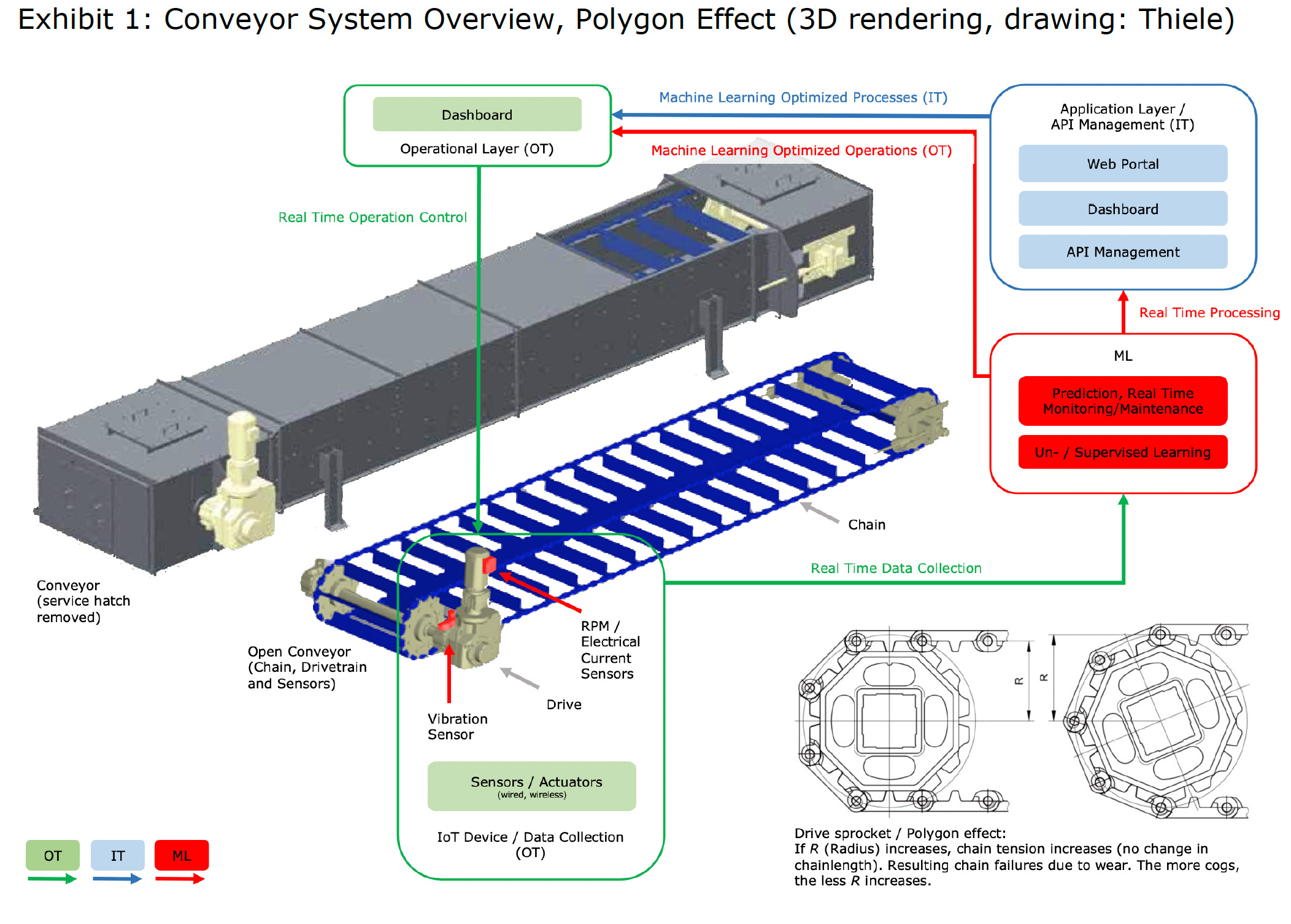
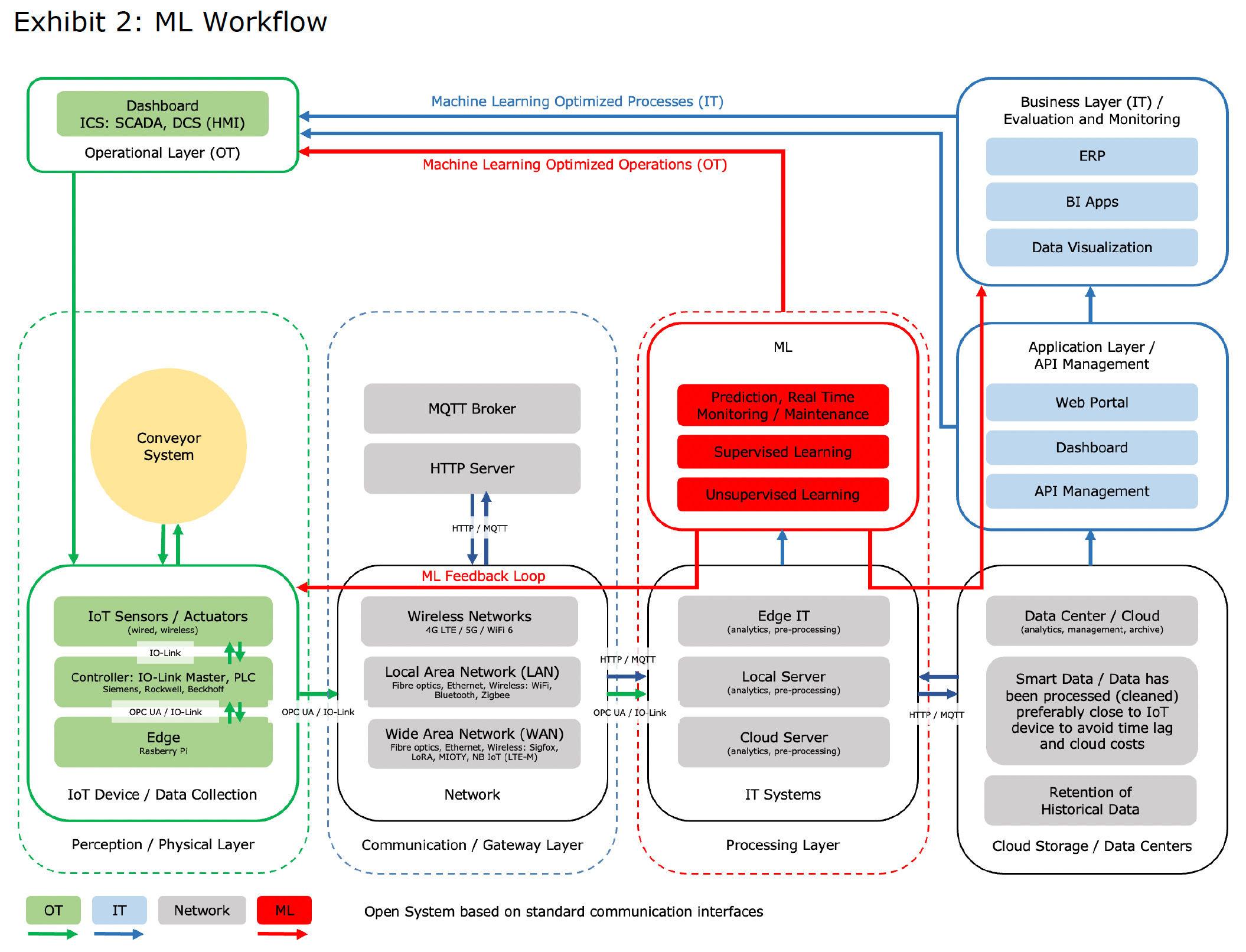